Decision stump
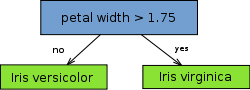
A decision stump is a machine learning model consisting of a one-level decision tree.[1] That is, it is a decision tree with one internal node (the root) which is immediately connected to the terminal nodes (its leaves). A decision stump makes a prediction based on the value of just a single input feature. Sometimes they are also called 1-rules.[2]
Depending on the type of the input feature, several variations are possible. For nominal features, one may build a stump which contains a leaf for each possible feature value[3][4] or a stump with the two leaves, one of which corresponds to some chosen category, and the other leaf to all the other categories.[5] For binary features these two schemes are identical. A missing value may be treated as a yet another category.[5]
For continuous features, usually, some threshold feature value is selected, and the stump contains two leaves — for values below and above the threshold. However, rarely, multiple thresholds may be chosen and the stump therefore contains three or more leaves.
Decision stumps are often[6] used as components (called "weak learners" or "base learners") in machine learning ensemble techniques such as bagging and boosting. For example, a state-of-the-art Viola–Jones face detection algorithm employs AdaBoost with decision stumps as weak learners.[7]
The term "decision stump" was coined in a 1992 ICML paper by Wayne Iba and Pat Langley.[1][8]
References
- 1 2 Iba, Wayne; and Langley, Pat (1992); Induction of One-Level Decision Trees, in ML92: Proceedings of the Ninth International Conference on Machine Learning, Aberdeen, Scotland, 1–3 July 1992, San Francisco, CA: Morgan Kaufmann, pp. 233–240
- ↑ Holte, Robert C. (1993). "Very Simple Classification Rules Perform Well on Most Commonly Used Datasets". CiteSeerX 10.1.1.67.2711
.
- ↑ Loper, Edward L.; Bird,, Steven; Klein, Ewan (2009). Natural language processing with Python. Sebastopol, CA: O'Reilly. ISBN 0-596-51649-5.
- ↑ This classifier is implemented in Weka under the name
OneR
(for "1-rule"). - 1 2 This is what has been implemented in Weka's
DecisionStump
classifier. - ↑ Reyzin, Lev; and Schapire, Robert E. (2006); How Boosting the Margin Can Also Boost Classifier Complexity, in ICML′06: Proceedings of the 23rd international conference on Machine Learning, pp. 753-760
- ↑ Viola, Paul; and Jones, Michael J. (2004); Robust Real-Time Face Detection, International Journal of Computer Vision, 57(2), 137–154
- ↑ Oliver, Jonathan J.; and Hand, David (1994); Averaging Over Decision Stumps, in Machine Learning: ECML-94, European Conference on Machine Learning, Catania, Italy, April 6–8, 1994, Proceedings, Lecture Notes in Computer Science (LNCS) 784, Springer, pp. 231–241 ISBN 3-540-57868-4 doi:10.1007/3-540-57868-4_61
Quote: "These simple rules are in effect severely pruned decision trees and have been termed decision stumps [cites Iba and Langley]".